
Scientific & Technological (Ai Based) Advancement In Pharmaceutical R&D & Clinical Trials

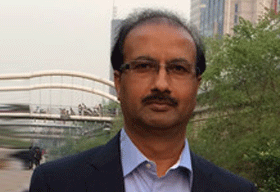
Artificial Intelligence (AI) is the ability of a computer or machine to simulate human intelligence and is dependent on three elements: massive amounts of data, sophisticated algorithms, and high-performance parallel processors. It is a broad term encompassing various ways in which computers process and find pat-terns within complex information and generate inferences or predictions from that data.
The idea of using artificial intelligence (AI) to accelerate drug discovery process and boost a success rate of pharmaceutical research programs has inspired a surge of activity in this area over the last several years. It can be applied to various types of healthcare data (structured and unstructured). The increasing availability of healthcare data and rapid development of big data analytic methods has made possible the recent successful applications of AI in healthcare.
With the ability to find hidden and unintuitive patterns in vast amounts of data in ways that no human can do, AI represents a considerable promise to transform many industries, including pharma and biotech.
AI in Various Phases of R& D
With more comprehensive understanding of the biological basis of disease through AI, the R&D process will be more streamlined to develop therapies from the offset, with optimal trial and errors. Reducing the failure rate will in turn save the industry billions of dollars.
AI for analyzing research literature, publications, and patents
AI-driven supercomputer for accelerating analysis and tests of hypotheses by researchers using ‘massive volumes of disparate data sources’ that include million sources of laboratory data reports as well as medical literature. AI is helpful for scientific data mining, data contextualization and deriving hypotheses. Navigating through all this information to draw meaningful insights about drug candidates is where AI-based algorithms become indispensable.
AI in Drug Discovery & Development
Application of big data analytics and machine learning-based AI for drug development thus augments human intelligence for analyzing data and identifying new patterns and insights It recognizes protein and ligand structures & interactions. Further, it can model bioactivity of small molecules & chemical interactions, and identify new molecules for the targets with previously unknown modulators.
Deep-learning screening of biomarkers includes genome, proteome, metabolome, and lipidome data of the biological samples to unravel the complex biological networks playing roles in diseases and help identify medications for specific patient populations. AI tool is used to identify molecular signatures and potential biomarkers for assessing the vaccine immunological response.
Computational physics and artificial intelligence for accurate molecular modeling of drug-like small molecules.(1) Neural network application is being applied for structure-based drug design and discovery in which researcher uses the application by virtually combining atoms to come-up with possible molecules.
Deep Genomics, a Toronto company, looks for patterns in genomic data to find causal relationships with specific diseases. BERG Health is using AI to analyze tissue samples, genomics, and other data pertinent to a disease, which has resulted in a potential new drug for topical squamous-cell carcinoma.
AI in Drug manufacturing
Through integration with AI self-learning machines, the complex operations in pharma manufacturing plants can be simplified to a greater degree. While this will certainly reduce the time and increase the efficiency, it will also enhance the reporting quality.
These developing technologies will enable operations to become intelligent and efficient, although they pose a challenge for the policy
makers and regulators to redefine the way we understand the current good manufacturing practices (cGMP).
AI-enabled machines will emphasize a means of rejecting the root-cause(s) for the output going ‘Out-of-Specification’. Understanding the logics & patterns, predicting the variations and adjusting the process beforehand will preempt unnecessary product failures. This will also minimize any redundancy in the process, improve the yield, ensure consistency, stabilize quality, and ultimately exhibit complete regulatory compliance, resulting in a product fulfilling its critical quality attributes. Thus, the expectations of the regulatory agencies in regards continuous quality verification (CQV) will evidently be satisfied by AI and its applications through machine learning tools.
AI in Clinical Trial Management
Artificial intelligence (AI) technology, combined with big data hold the potential to solve many key clinical trial challenges with a better protocol design, patient enrolment and retention, and study start-up as prime areas for improvement.
Data-driven strategies powered by advanced AI algorithms processing data collected from mobile sensors and apps, electronic medical and administrative records, and other sources have the potential to reduce trial costs.
AI In Healthcare
AI in healthcare is currently geared towards improving patient outcomes, aligning the interests of various stake-holders and reducing healthcare costs. It is beginning to play a role in quantifying the quality of service patients receive at hospitals.
AI in Diagnostic Areas
Artificial intelligence is turning the smartphone and consumer wearables into powerful at-home diagnostic tools. AI-chatbots are being used with speech recognition capability to identify patterns in patient symptoms to form a potential diagnosis, prevent disease and/or recommend an appropriate course of action. The AI assistant can instantly provide information on the patient's past & present health and make suggestions that would help in the diagnosis.
Google has developed an AI eye doctor, trained to report leaky and fragile blood vessels as symptoms of poorly controlled diabetes. China-based Infervison has developed an AI system with GE Healthcare, Cisco, and Nvidia that is trained to analyze computerized tomography (CT) scans and X-rays to detect lesions and nodules that could be early symptoms of lung cancer. If we could automate the diagnosis, doctors would be able to spend a lot more time with their patients or could attend to more patients, leading to better healthcare delivery.
Robotic Assistance
Artificial intelligence guiding the hand of the surgeon through the help of robots, opens the doors to extremely high levels of precision and better patient outcomes. Highly precise movements are made by the robot hands. So, any tremors in the surgeon's hands will be neutralized completely, enabling the progress and success of micro-surgeries followed by continuous post-surgery monitoring. All this can have huge implications on hospital stay, and thereby recovery of patient.
Proactive Medical Care
With Artificial Intelligence, there has been a shift in this trend because reactive medical care became proactive medical care. The patient's complete medical history is studied and high-risk markers for various diseases are highlighted. There are apps that drive the patient to be an active participant in their own health scenario. Similarly, there are condition-specific applications for AI like palliative care, congenital heart diseases and diabetes management.
Challenges with AI
Despite its potential to unlock new insights and streamline the way providers and patients interact with healthcare data, AI may bring not inconsiderable threats of privacy problems, ethics concerns, and medical errors. Trust and accuracy are only (a big) part, while whole new set of challenges revolve around data privacy and security - challenges that are compounded by the fact that most algorithms need access to massive datasets for training and validation. Balancing data with regulatory needs is an industry challenge.
Concluding Remarks
AI now features in our daily lives: as virtual assistants on our smartphones, within our Google searches and translations, and for sure in our self-driving cars and home-help robots sooner or later.
The key enablement factor of the future ‘AI-driven revolution’ in pharmaceutical research is data. AI focused on organizing and structuring healthcare data for deriving actionable insights for the design and interpretation of clinical trials. Clinical trial data, claims, pharmacy history, electronic health records and genomics data on patients to accelerate discovery and development of new therapies.
The major benefits enjoyed by both the patient and medical practitioners are predictive medical care, personalized medication, better diagnosis, advanced treatment plans, non-stop monitoring means lower liability for hospital, long term cost savings for both patient and medical care provider.
Ensuring that artificial intelligence develops ethically, safely, and meaningfully in healthcare will be the responsibility of all stakeholders. Balancing the risks and rewards of AI in healthcare will require collaborative effort from technology developers, regulators, end-users, and consumers.
AI-enabled machines will emphasize a means of rejecting the root-cause(s) for the output going ‘Out-of-Specification’. Understanding the logics & patterns, predicting the variations and adjusting the process beforehand will preempt unnecessary product failures. This will also minimize any redundancy in the process, improve the yield, ensure consistency, stabilize quality, and ultimately exhibit complete regulatory compliance, resulting in a product fulfilling its critical quality attributes. Thus, the expectations of the regulatory agencies in regards continuous quality verification (CQV) will evidently be satisfied by AI and its applications through machine learning tools.
AI in Clinical Trial Management
Artificial intelligence (AI) technology, combined with big data hold the potential to solve many key clinical trial challenges with a better protocol design, patient enrolment and retention, and study start-up as prime areas for improvement.
Technologies such as digital reporting apps as well as wearables, allow for real-time engagement, communication, and support patient-centric trials. Patients can send feedback on treatment symptoms, manage medication intake and can share information with researchers with more meaningful clinically relevant insights and be used to assess and develop trial objectives, endpoints and procedures, reducing or eliminating the need for patients to travel to sites, which increases patient adherence and compliance. AI analysis of live remote data also can detect when patients may not be compliant, allowing clinical personnel to intervene before a patient's data must be excluded.With Artificial Intelligence, there has been a shift in this trend because reactive medical care became proactive medical care
Data-driven strategies powered by advanced AI algorithms processing data collected from mobile sensors and apps, electronic medical and administrative records, and other sources have the potential to reduce trial costs.
AI In Healthcare
AI in healthcare is currently geared towards improving patient outcomes, aligning the interests of various stake-holders and reducing healthcare costs. It is beginning to play a role in quantifying the quality of service patients receive at hospitals.
AI in Diagnostic Areas
Artificial intelligence is turning the smartphone and consumer wearables into powerful at-home diagnostic tools. AI-chatbots are being used with speech recognition capability to identify patterns in patient symptoms to form a potential diagnosis, prevent disease and/or recommend an appropriate course of action. The AI assistant can instantly provide information on the patient's past & present health and make suggestions that would help in the diagnosis.
Google has developed an AI eye doctor, trained to report leaky and fragile blood vessels as symptoms of poorly controlled diabetes. China-based Infervison has developed an AI system with GE Healthcare, Cisco, and Nvidia that is trained to analyze computerized tomography (CT) scans and X-rays to detect lesions and nodules that could be early symptoms of lung cancer. If we could automate the diagnosis, doctors would be able to spend a lot more time with their patients or could attend to more patients, leading to better healthcare delivery.
Robotic Assistance
Artificial intelligence guiding the hand of the surgeon through the help of robots, opens the doors to extremely high levels of precision and better patient outcomes. Highly precise movements are made by the robot hands. So, any tremors in the surgeon's hands will be neutralized completely, enabling the progress and success of micro-surgeries followed by continuous post-surgery monitoring. All this can have huge implications on hospital stay, and thereby recovery of patient.
Proactive Medical Care
With Artificial Intelligence, there has been a shift in this trend because reactive medical care became proactive medical care. The patient's complete medical history is studied and high-risk markers for various diseases are highlighted. There are apps that drive the patient to be an active participant in their own health scenario. Similarly, there are condition-specific applications for AI like palliative care, congenital heart diseases and diabetes management.
Challenges with AI
Despite its potential to unlock new insights and streamline the way providers and patients interact with healthcare data, AI may bring not inconsiderable threats of privacy problems, ethics concerns, and medical errors. Trust and accuracy are only (a big) part, while whole new set of challenges revolve around data privacy and security - challenges that are compounded by the fact that most algorithms need access to massive datasets for training and validation. Balancing data with regulatory needs is an industry challenge.
Concluding Remarks
AI now features in our daily lives: as virtual assistants on our smartphones, within our Google searches and translations, and for sure in our self-driving cars and home-help robots sooner or later.
The key enablement factor of the future ‘AI-driven revolution’ in pharmaceutical research is data. AI focused on organizing and structuring healthcare data for deriving actionable insights for the design and interpretation of clinical trials. Clinical trial data, claims, pharmacy history, electronic health records and genomics data on patients to accelerate discovery and development of new therapies.
The major benefits enjoyed by both the patient and medical practitioners are predictive medical care, personalized medication, better diagnosis, advanced treatment plans, non-stop monitoring means lower liability for hospital, long term cost savings for both patient and medical care provider.
Ensuring that artificial intelligence develops ethically, safely, and meaningfully in healthcare will be the responsibility of all stakeholders. Balancing the risks and rewards of AI in healthcare will require collaborative effort from technology developers, regulators, end-users, and consumers.